Deepfake videos have been around for the past couple of years and some have been finding some fun in it by manipulating movie and TV scenes with different actors, but such manipulation of real-life footage via artificial intelligence could also be extremely dangerous, so deepfake detectors have been incredibly helpful in fact-checking and taking down doctored videos.
However, it now turns out that such tools could be defeated, according to computer scientists from the University of California, San Diego.
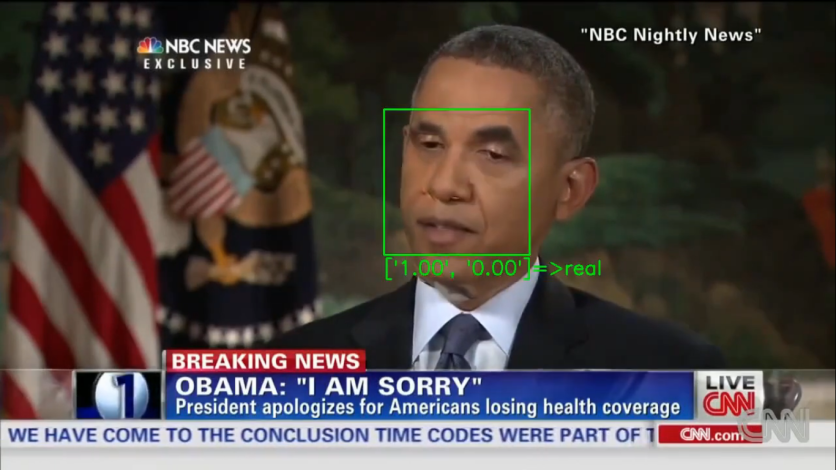
The Use of 'Adversarial Examples'
In a report by News18, deepfake detectors could be fooled by adding inputs known as "adversarial examples," which are slightly manipulated inputs, into every video frame.
The adversarial examples cause artificial systems like machine learning models to make mistakes, thinking that the deepfaked videos have never been touched, and according to the UC San Diego scientists, that the attack could still work despite compressing the fake video, which should be able to remove false features.
Basically, detectors can determine real videos from fake ones by focusing on facial features, such as the eye movements, which are commonly poorly reproduced in manipulated videos.
Unfortunately, this method could trick even the most sophisticated detectors available.
The alarming evidence was showcased during the last Winter Conference on Applications of Computer Vision (WACV) 2021 that was held on January 5 to January 9, 2021.
'Could be Real-World Threat'
"Our work shows that attacks on deepfake detectors could be a real-world threat," said Shehzeen Hussain, a computer engineering Ph.D. student at UC San Diego and the first co-author of the WACV paper, in the UCSD Jacobs School of Engineering online newspaper.
"More alarmingly, we demonstrate that it's possible to craft robust adversarial deepfakes in even when an adversary may not be aware of the inner workings of the machine learning model used by the detector," Hussain further said.
According to the report, the scientists were able to defeat the vigilance of even the most sophisticated detectors even without access to the detector model when they injected the adversarial examples.
High Success Rates
To test the attacks, the researchers created two scenarios: one where they have complete access to the detector model, including the architecture and parameters of the classification model and the face extraction pipeline; and another where they can only query the machine.
In the first scenario, the scientists had a 99% success rate in uncompressed video and 84.96% for the compressed ones.
For the second scenario, their success rate was 86.43% for uncompressed videos and 78.33% for the compressed files.
The scientists said this is the first time evidence has been shown that detectors can be defeated.
With that, the scientists behind the paper are encouraging improved training of the software to better detect such manipulations, recommending an approach that is similar to what they call as adversarial training, wherein an adaptive adversary continuously generates new deepfakes while detectors continue to learn to detect the manipulated samples.
They have also declined to release the codes they used so as not to be used by hostile parties.
Related Article : Beware of TikTok Fake Stock, Financial Advice! How to Avoid Getting Fooled; Best Advisor Websites
This article is owned by Tech Times
Written by: Nhx Tingson