Mind reading has come one step closer towards reality as scientists have recently developed a new artificial intelligence that can turn brain activity into text! A.I. developers and scientists have actively been working on understanding the human brain as a reference for A.I. technology, and now, scientists have gotten closer by becoming able to turn thoughts into text!
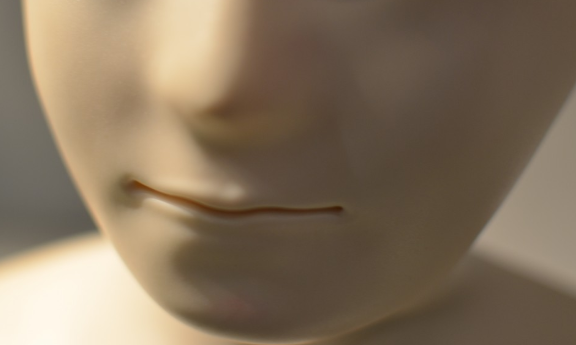
The AI's system
Currently, the system is working on neutral patterns being detected while someone is speaking in a loud manner. Experts said this could eventually aid communication for the patients who are unable to speak as those with locked in syndrome.
According to Dr. Joseph Makin, co-author of this specific research from the University of California located in San Francisco, "We are not there yet, but we think this could be the basis of a speech prosthesis."
How does this system work?
Makin and colleagues wrote in the journal Nature Neuroscience about how they were able to develop their own system by recruiting four different patients who have had electrode arrays implanted in their brains in order to monitor the epileptic seizures.
The participants were then made to read aloud from about 50 set sentences multiple times, including sentences like "Tina Turned a pop singer," as well as "Those thieves stole 30 jewels". The team was then able to track their neutral activity while the words were being spoken.
The data was then processed by a machine-learning algorithm, which is a type of artificial intelligence system that is able to convert brain activity data for the spoken sentences into a series of numbers.
In order to secure the validity of the numbers, the system then compared the sounds predicted from the little pieces of brain activity data together with the actual recorded audio. The numbers are then processed into the second part of the system responsible for converting the data into a series of words.
How did the system turn out?
First, the system was not able to say anything but gibberish, but as the system started to compare each sequence of words together with the actual sentences that had been read aloud, it was able to improve. The machine then learned which numbers were related to words and which words were most likely to follow each other.
The team was able to find out that the accuracy of the new system was much better than the old one. The accuracy level was different from person to person, and for one person, only 3% of his sentences needed to be corrected, which the word error rate for professional human transcribers is only at 5%.
According to Makin, "If you try to go outside the [50 sentences used] the decoding gets much worse," which indicates that the AI has a lot of improvements to undergo before it can be deemed as reliable. Another problem noted was that the system relied on the brain activity of those people who actively speak a sentence out loud, which could mean that this may differ from people who do not actively speak.