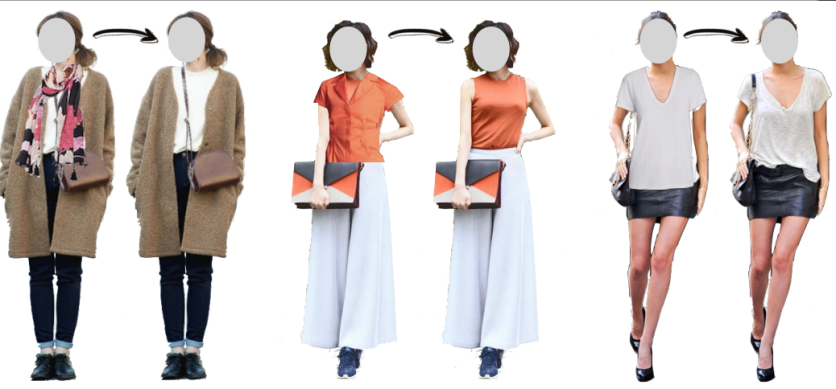
Researchers from the University of Texas at Austin (UT-Austin), Cornell Tech, Georgia Tech, and Facebook AI Research have created an artificial intelligence system that can be used to give personalized fashion advice, published here.
Now one of the projects under UT-Austin's Department of Computer Science, Fashion++, was created with a philosophy of making minimal changes to maximize outfit fashionability. According to a press release from the UT-Austin, the system analyzes several factors of outfits, such as the color, pattern, texture, and shape, and gives recommendations based on this analysis.
How Fashion++ works
The researchers posted a video summarizing how Fashion++ works. A key component of how the system works is in machine learning. Using thousands of outfit images posted by fashion enthusiasts, Fashion++ was trained to learn the components of a fashionable outfit. Likewise, by mashing up several parts of these outfits, the artificial intelligence system was able to determine what an unfashionable outfit looked like.
In the first phase, called encoding, the system breaks down an image's outfit regions (e.g. top and bottom) into its key components, such as shape and texture. From there, a code is applied to translate it into a way the system understands.
The next phase, called editing, sees the system analyzing the data to make recommendations that would improve an outfit's fashionability, translated into an edited set of codes.
The last phase, called decoding, uses the edited codes to generate these recommendations onto the existing image. This way, the user can visualize the effects of making minor edits to their outfit virtually (as opposed to digging through their closet and trying it on in real life).
The Fashion++ system can generate several suggestions depending on your desired outcome (either least edits or most fashionability).
Fashion++: is it effective?
By using both quantitative measurements and human evaluation, the system was able to generate recommendations that improve fashionability with the least number of edits. By being able to visualize these recommendations through Fashion++'s rendered images, users can easily see the impact of adding or removing an accessory, changing the layering of outfits, or even tucking in a shirt, all without the hassle of having to do it in real life.
There are still some improvements to be made in the system, such as factoring in different body sizes and shapes, improving fashion representation from different cultures all over the world, and analyzing fashionable outfits prior to the 90s (accounting for style changes over the decades).
The researchers are exploring ways to further the Fashion++ system. Aside from looking at more training sources, like Instagram, for example, they're also looking at ways to make more personalized suggestions for each user. This would include factoring in the personal style of the user as well as what's already in that user's wardrobe.
Prof. Kristen Grauman, one of the authors of the study, shares her sentiments on the future of Fashion++, shared in a press release from UT-Austin:
"We are examining the interaction between how a person's body is shaped and how the clothing would suit them. We're excited to broaden the applicability to people of all body sizes and shapes by doing this research."